Elevating Business through Machine Learning Data Labeling
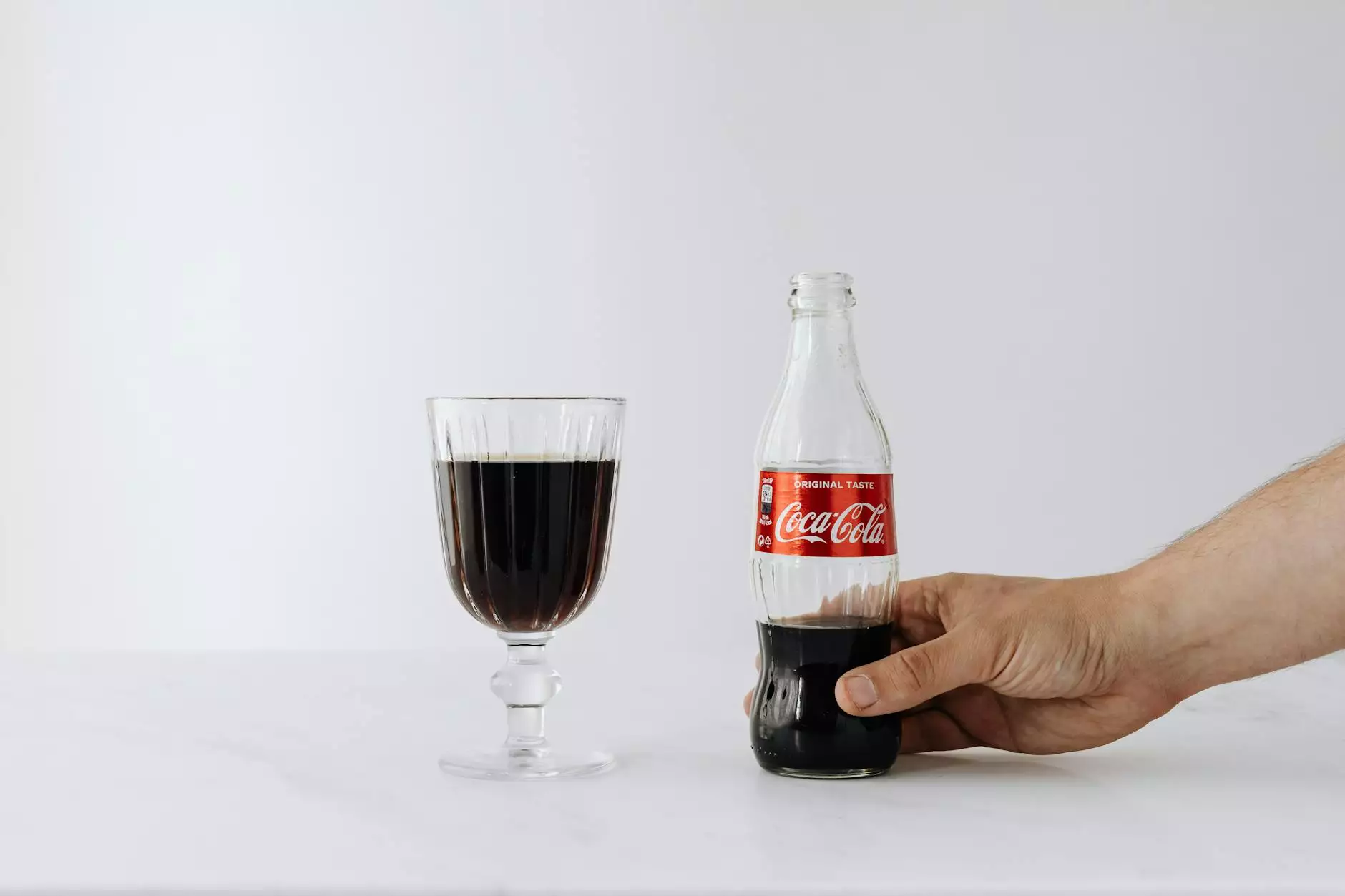
In an era where digital transformation is not just a choice but a necessity, the role of machine learning data labeling has become increasingly significant. Businesses across various sectors are leveraging this technology to gain insights, enhance efficiency, and drive decision-making. This article explores the intricacies of machine learning data labeling and how it can transform your business operations, specifically in the realm of home services, keys, and locksmiths.
Understanding Machine Learning Data Labeling
Machine learning data labeling involves the process of identifying and annotating raw data to train machine learning models. Essentially, it helps machines understand the context of the data they analyze. For businesses, especially in home services, this means being able to automate repetitive tasks, streamline operations, and ultimately improve customer satisfaction.
The Importance of Data in Machine Learning
Data is the lifeblood of machine learning. Without high-quality data, the efficacy of machine learning models diminishes significantly. Data labeling serves to ensure that the models learn from the most accurate information available. The following points highlight why data labeling is crucial:
- Clarity: Clear annotations help machines interpret data correctly, leading to more accurate predictions.
- Contextual Understanding: Labeling provides context, allowing algorithms to grasp subtle nuances in data.
- Enhanced Performance: Well-labeled data generally results in improved model performance and reliability.
How Machine Learning Data Labeling Enhances Business Operations
For businesses in the home services and locksmith industry, machine learning data labeling can yield substantial benefits. Here are key areas where it can make a difference:
1. Improving Customer Interactions
Customer experience is paramount in service industries. Machine learning algorithms, powered by labeled data, can help businesses personalize their interactions. By analyzing customer data, businesses can:
- Predict customer preferences for keys and home services.
- Offer personalized recommendations based on previous interactions.
- Automate responses to common inquiries, freeing up time for staff to handle complex tasks.
2. Efficient Inventory Management
Another significant application of machine learning data labeling in the locksmith business is in inventory management. By analyzing sales data and labeling it accordingly, businesses can:
- Forecast demand for specific types of keys and locks.
- Minimize overstock and stockouts, leading to cost savings.
- Optimize supply chain processes, ensuring timely restocking of essential items.
3. Streamlining Operations
Machine learning data labeling can streamline various operational aspects. For instance, companies can automate scheduling, resource allocation, and compliance checks based on reliable data labels. This leads to:
- Reduced operational costs.
- Enhanced service delivery efficiency.
- Improved compliance with industry regulations.
4. Accurate Market Analysis
Market analysis is fundamental for any business's strategic planning. By using labeled data, companies in the home services and locksmith sectors can:
- Identify market trends and consumer behavior patterns.
- Gauge the competition effectively.
- Make informed decisions on service offerings and pricing strategies.
Data Labeling Techniques for Effective Machine Learning
There are various techniques employed in machine learning data labeling. Understanding these can help businesses choose the right approach to leverage their data effectively:
1. Manual Labeling
Manual labeling involves humans annotating the data directly. This approach is beneficial for:
- Ensuring high accuracy levels, especially in complex tasks.
- Providing context that automated tools might miss.
- Developing specialized datasets tailored to specific business needs.
2. Automated Labeling
Automated data labeling uses algorithms to annotate data based on predetermined rules. This method can:
- Save time and resources, allowing companies to process large datasets quickly.
- Be cost-effective for bulk data labeling projects.
- Complement manual efforts by handling initial labeling tasks.
3. Semi-Automatic Labeling
This technique combines both manual and automated efforts. It is particularly useful as it:
- Enhances efficiency without sacrificing accuracy.
- Reduces the workload on human annotators while still allowing for oversight and quality control.
- Can be tailored to fit specific project requirements and data types.
Challenges in Machine Learning Data Labeling
While the advantages are substantial, machine learning data labeling comes with its challenges. Businesses must recognize and address these challenges to fully leverage the potential of their data:
1. Quality Control
Ensuring the accuracy of labeled data is critical. Inaccuracies can propagate through machine learning models, leading to faulty outputs. Implementing rigorous quality checks is essential.
2. Scalability
As businesses grow, the volume of data can become overwhelming. A scalable data labeling strategy is pivotal to manage this increase in data while still maintaining quality.
3. Cost Management
Data labeling can be resource-intensive. Companies need to determine an appropriate budget and invest strategically in tools or personnel to maximize return on investment.
The Future of Machine Learning Data Labeling in Business
The future of machine learning data labeling appears promising. As technology continues to evolve, several trends will likely shape its trajectory:
- Increased Automation: The trend toward automation in data labeling is expected to grow, enabling faster processing and scalability.
- AI-Driven Labeling Solutions: As artificial intelligence advances, we can anticipate the development of more sophisticated labeling tools that require minimal human intervention.
- Collaborative Labeling Platforms: Businesses may increasingly turn to collaborative platforms where teams can work together to label data efficiently.
Conclusion
In summary, machine learning data labeling is poised to revolutionize the home services, keys, and locksmith industries. By harnessing the power of labeled data, businesses can enhance customer interactions, streamline operations, and make informed strategic decisions. Embracing this technology offers a competitive edge in a fast-evolving market landscape.
As we move forward, it's crucial for businesses to invest in effective data labeling strategies and recognize its importance not just as a technical task, but as a cornerstone of modern business intelligence.