Unlocking Business Potential with Machine Learning Data Labeling
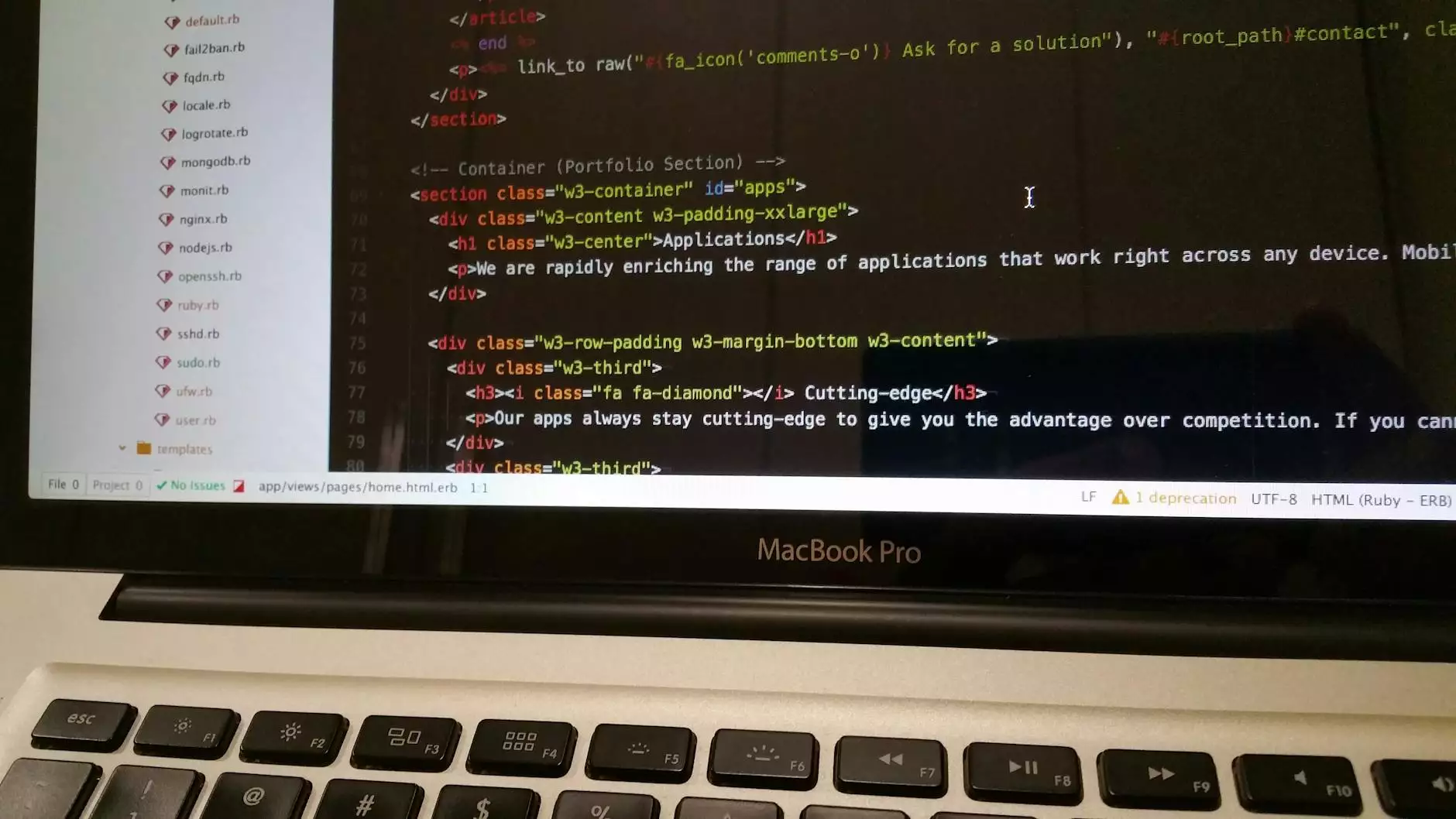
In the era of artificial intelligence and machine learning, data has become the lifeblood of numerous industries. With the exponential growth of data, the demand for accurate and efficient data labeling has surged, paving the way for a new norm in business operations. This article delves deep into the world of machine learning data labeling, emphasizing its crucial role in enhancing business capabilities.
Understanding Machine Learning Data Labeling
Machine learning data labeling involves the process of annotating data sets to produce labeled data that can be used to train machine learning models. The objective is to provide machine learning systems with the necessary input so they can learn to make predictions or classifications based on that data. Properly labeled data is essential for the accuracy and effectiveness of any machine learning project.
The Importance of Data Labeling in Machine Learning
Data labeling is not just a technical task; it’s a fundamental step that influences the entire machine learning pipeline. Here’s why data labeling is critical:
- Enhances Model Performance: Well-labeled data helps algorithms learn more effectively, improving their accuracy and robustness.
- Reduces Error Rates: Accurate annotations lower the risks of misclassification in predictive models.
- Facilitates Automation: Automating processes can be achieved through well-structured labeled data, thereby increasing operational efficiency.
- Enables Insight Extraction: Labeled data allows organizations to draw meaningful conclusions from data analysis, driving better decision-making.
The Role of Data Annotation Tools and Platforms
Businesses today are leveraging advanced data annotation tools and platforms to streamline their data labeling processes. These tools are designed to help teams manage large volumes of data efficiently while ensuring consistent annotation quality.
Key Features of High-Quality Data Annotation Tools
- User-Friendly Interface: Intuitive design helps reduce training time and enhances productivity.
- Scalability: The ability to handle increasing volumes of data is critical as businesses grow.
- Multi-Type Annotation Support: Tools should support various data types, including images, videos, and text.
- Collaboration Features: Built-in functionalities for teams to work together effectively can significantly boost productivity.
- Quality Control Mechanisms: Ensuring the accuracy of annotations through validation steps is vital for success.
Keylabs.ai: Pioneering the Future of Data Annotation
Among the industry leaders in machine learning data labeling is Keylabs.ai. Their comprehensive data annotation platform offers a range of functionalities designed to meet the diverse needs of different sectors.
Keylabs.ai Services
- Customized Data Annotation: Tailored solutions to address specific project requirements.
- Expert Annotator Teams: Skilled professionals ensuring high-quality annotations.
- Automated and Manual Options: Flexibility in choosing between automated tools or hands-on approaches.
- Industry-Specific Solutions: Specialized services for healthcare, automotive, retail, and more.
Real-World Applications of Machine Learning Data Labeling
The significance of machine learning data labeling can be seen across various industries. Here are some notable applications:
1. Healthcare
In the healthcare sector, labeled data play a critical role. It is used in medical imaging to identify diseases, in radiology for diagnosis, and even in epidemiology for predicting disease outbreaks. Accurate labeling leads to better training of diagnostic models, improving patient care and outcomes.
2. Autonomous Vehicles
Self-driving cars rely on vast amounts of labeled data to understand their surroundings. From identifying pedestrians to interpreting traffic signs, accurate data labeling is crucial for enhancing the safety and reliability of autonomous driving technologies.
3. Retail and E-commerce
In retail, machine learning algorithms help in understanding consumer behavior, managing inventory, and personalizing shopping experiences. Labeled data enables these algorithms to recognize patterns and predict trends, leading to improved marketing strategies and customer satisfaction.
4. Finance
Financial institutions utilise machine learning to detect fraudulent transactions and assess credit risk. By labeling historical transaction data, banks can train models that accurately flag unusual behavior, thus safeguarding against fraud and improving customer trust.
How to Optimize Your Data Labeling Process
Establishing an efficient data labeling workflow can greatly impact the success of your machine learning initiatives. Consider the following best practices:
1. Clearly Define Objectives
Before starting the data labeling process, define your goals and the types of outputs you expect from your machine learning model. Clear objectives lead to more focused data collection and labeling efforts.
2. Choose the Right Tools
Invest in robust data annotation tools that can streamline the labeling process. Tools that offer automation, quality control features, and an intuitive user experience will save time and enhance quality.
3. Implement Quality Assurance Procedures
Integrate checks and reviews throughout the labeling process to catch errors early. Regular audits and feedback loops can help maintain a high standard of data quality.
4. Train Your Team
Ensure that team members involved in the labeling process are well-trained. Educating annotators about the nuances of your specific domain can significantly improve the quality of work produced.
5. Collaborate and Iterate
Data labeling is an iterative process. Foster collaboration among team members, encourage open dialogue about challenges faced, and be willing to adapt and improve over time.
Conclusion
Machine learning data labeling is more than just marking data points; it is an essential driver of innovation and efficiency in today’s digitally-driven business environment. By leveraging advanced data annotation tools and platforms such as Keylabs.ai, organizations can unlock the full potential of their data, leading to enhanced decision-making and ultimately, greater business success. As the demand for intelligent systems continues to rise, mastering the art of data labeling will remain a top priority for businesses striving to maintain a competitive edge.
In conclusion, investing time and resources in effective data labeling strategies is not just beneficial; it is imperative for any organization looking to thrive in an increasingly data-centric landscape.
© 2023 Keylabs AI. All rights reserved.